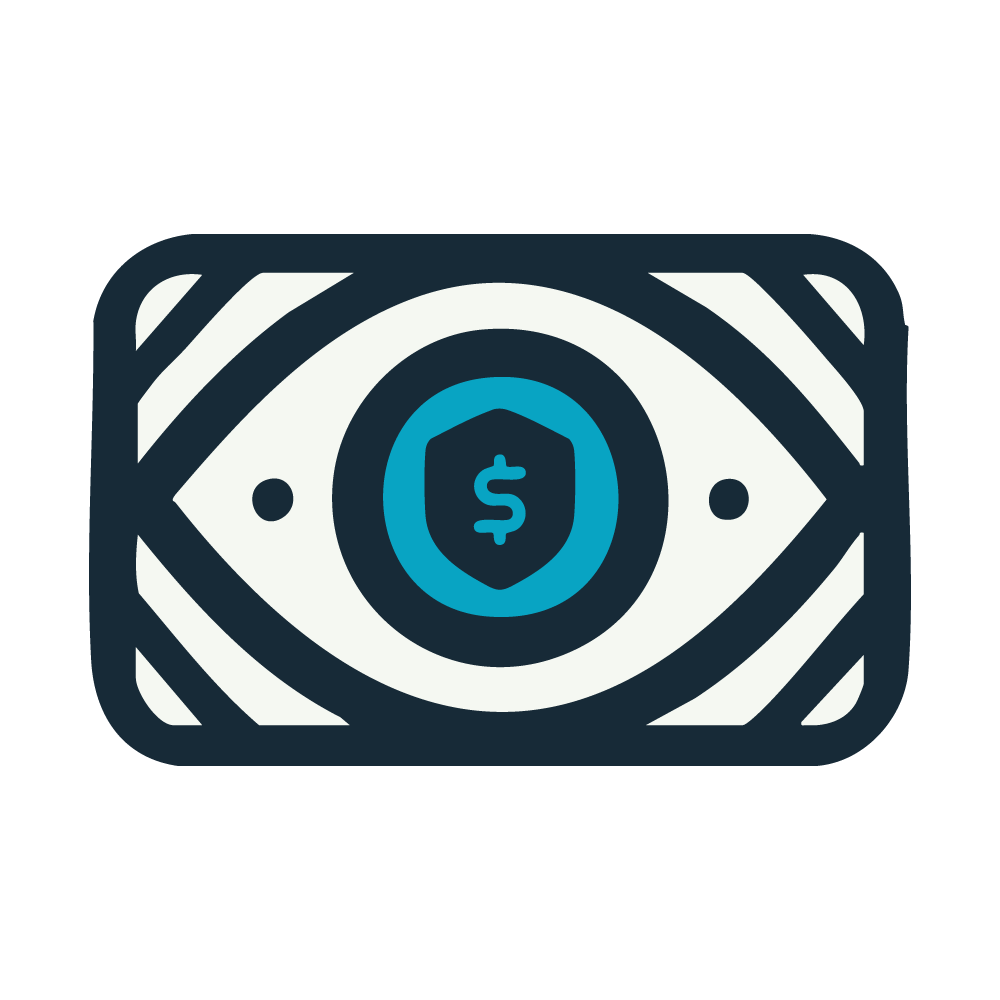
RiskSentry ML
Project Overview
RiskSentry ML is a demonstration project showcasing practical machine learning implementation for transaction risk analysis. Built as a learning exercise, it implements a Random Forest Classifier model through a Flask API, demonstrating the complete workflow from data processing to model deployment.
Core ML
Random Forest
Feature Engineering
Pattern Detection
Implementation
Flask API
Real-time Processing
Interactive UI
Data Focus
Transaction Analysis
Risk Scoring
Pattern Recognition
Risk Assessment Interface
Transaction Analysis
Comprehensive risk analysis with multiple detection patterns:
Risk Patterns
- Location anomalies
- Time-based risks
- Amount patterns
- Transaction combinations
Detection Features
- High-risk country detection
- Night transaction analysis
- Suspicious amount ranges
- Pattern correlation
Model Analytics
Performance Metrics
- Cross-validation scores
- Feature importance
- Confusion matrix
- ROC curves
Real-time Monitoring
- Prediction confidence
- Processing latency
- Model drift detection
- Error tracking
Technical Architecture
ML Pipeline
- Model: Random Forest Classifier
- Features: 15 engineered features
- Processing: Real-time scoring
- Validation: Cross-validation setup
API Layer
- Framework: Flask with REST endpoints
- Processing: Async prediction handling
- Security: Request validation
- Format: JSON response structure
Implementation Details
Feature Engineering
- Time-based pattern extraction
- Transaction amount analysis
- Location risk scoring
- Behavioral pattern detection
Model Development
- Synthetic data generation
- Hyperparameter optimization
- Cross-validation implementation
- Performance metrics tracking
Data Processing Pipeline
Feature Engineering
- Time-based feature extraction
- Geographic risk analysis
- Amount pattern detection
- Transaction type classification
Model Processing
- Random Forest classification
- Multi-factor risk scoring
- Pattern correlation analysis
- Real-time prediction pipeline
Technical Implementation
Core Technologies
- Python with Flask web framework
- scikit-learn ML implementation
- NumPy/Pandas data processing
- Bootstrap responsive design
Machine Learning Pipeline
- Random Forest Classifier model
- Feature engineering system
- Pattern detection algorithms
- Probability-based scoring
API Architecture
- RESTful endpoint design
- CORS security configuration
- Input validation system
- Error handling protocols
Data Engineering
- Synthetic data generation
- Pattern simulation
- Time series processing
- Data normalization
Performance Features
- Memory optimization
- Response time tuning
- Resource management
- Efficient data handling
Testing Framework
- Unit test implementation
- API endpoint validation
- Pattern detection testing
- Response verification
Code Implementation Highlights
Pattern Detection System
Multi-factor risk analysis with weighted scoring:
# Complex pattern detection with weighted risk factors
pattern_risks = {
'location_anomaly': bool(df['location'].iloc[0] in ['RU', 'BR', 'CN', 'UK']),
'time_anomaly': bool(0 <= df['hour'].iloc[0] <= 5),
'amount_anomaly': bool(
df['amount'].iloc[0] < 10 or # Small amounts (card testing)
df['amount'].iloc[0] > 500 or # Large amounts
(df['amount'].iloc[0] >= 900 and df['amount'].iloc[0] <= 999.99) # Suspicious range
),
'transaction_type_risk': bool(
(df['transaction_type'].iloc[0] == 'atm' and 0 <= df['hour'].iloc[0] <= 5) or
(df['transaction_type'].iloc[0] == 'online' and df['amount'].iloc[0] < 10)
)
}
risk_weights = {
'location_anomaly': 1.5,
'time_anomaly': 1.0,
'amount_anomaly': 2.0,
'transaction_type_risk': 1.5
}
Feature Engineering
Complex risk scoring and indicator generation:
# Composite risk indicators with multiple factors
df['risk_score'] = (
((df['hour'] < 6).astype(int)) +
df['amount_999'].astype(int) * 2 +
df['amount_high'].astype(int) * 2 +
df['is_high_risk_location'].astype(int) * 3
)
df['card_testing_risk'] = (df['small_amount'] + df['high_velocity'] + df['is_online']) / 3
df['location_time_risk'] = (df['is_high_risk_location'] + (df['hour'] < 6).astype(int)) / 2
Synthetic Data Generation
Intelligent pattern-based test data creation:
def generate_fraudulent_pattern(user_profile):
patterns = [
# Card testing pattern
lambda: {
'amount': np.random.uniform(1, 5),
'location': np.random.choice(['UK', 'RU', 'BR', 'CN']),
'is_fraud': 1,
'is_card_testing': True
},
# After hours + location change
lambda: {
'amount': user_profile['avg_amount'] * np.random.uniform(3, 6),
'location': np.random.choice(['UK', 'RU', 'BR', 'CN']),
'timestamp': datetime.now().replace(hour=np.random.randint(1, 5)),
'is_fraud': 1
}
]
return np.random.choice(patterns, p=weights)()
Risk Display Algorithm
Intelligent probability normalization for UI:
// Intelligent risk display with normalized probability
const rawProbability = result.fraud_probability * 100;
let displayProbability;
if (rawProbability <= 11) {
// Normal range (0-11% maps to 0-30%)
displayProbability = (rawProbability / 11) * 30;
} else if (rawProbability <= 29) {
// Elevated range (12-29% maps to 31-70%)
displayProbability = 30 + ((rawProbability - 11) / 18) * 40;
} else {
// High risk range (30-36% maps to 71-100%)
displayProbability = 70 + ((rawProbability - 29) / 7) * 30;
}
Development Challenges
Model Training
Creating realistic synthetic training data
Solution:
- Generated balanced pattern sets
- Implemented varied risk scenarios
- Validated pattern distributions
Real-time Processing
Optimizing prediction response time
Solution:
- Implemented feature caching
- Optimized model loading
- Added response streaming
Credits
This project serves as a demonstration of machine learning applications in risk analysis. Built with scikit-learn for the ML components and Flask for the API layer, it showcases the potential of automated risk assessment in financial transactions.
Special thanks to the open-source community for the powerful libraries and tools that made this project possible. The project emphasizes the practical application of machine learning techniques for pattern recognition and risk analysis.