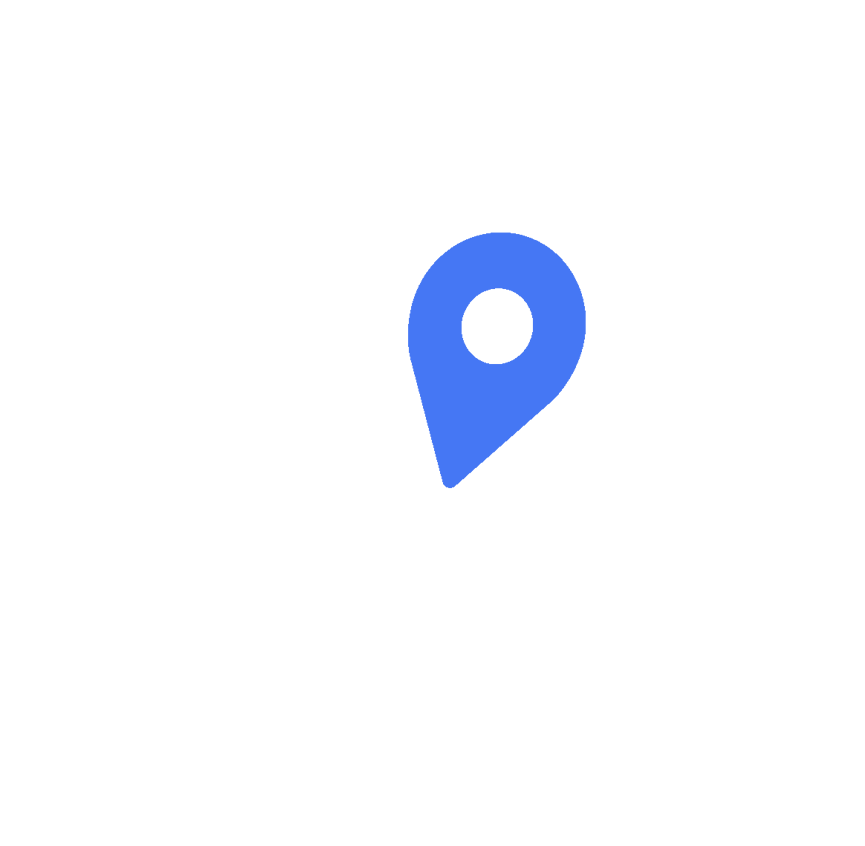
Bird Species ML Classifier
Case Study: Deep Learning Classification
Model Performance
91.23% Accuracy
117 Species
292,500 Images
Architecture
EfficientNetB3
Attention Layer
Transfer Learning
Training
48 Epochs
Mixed Precision
Gradient Clipping
This case study explores the development of a high-accuracy bird species classification model using deep learning techniques. The model achieves 91.23% accuracy across 117 species through careful architecture design, extensive data augmentation, and optimization strategies.
Development Objectives
Model Requirements
- High accuracy fine-grained classification
- Efficient inference for mobile deployment
- Robust to varying image conditions
Technical Goals
- Custom attention mechanism for detail focus
- Mixed precision training optimization
- Model size reduction for deployment
Data Processing Pipeline
Data Augmentation
- Random rotation (±30°)
- Random zoom (0.8-1.2x)
- Horizontal flips
- Color jittering
Image Preprocessing
- Resolution standardization (300x300)
- Normalization (-1 to 1 range)
- Background noise reduction
- Auto-contrast enhancement
Data Pipeline Implementation
class BirdDataPipeline:
def __init__(self, image_size=300, batch_size=32):
self.image_size = image_size
self.batch_size = batch_size
self.augmentation = tf.keras.Sequential([
tf.keras.layers.RandomRotation(0.2),
tf.keras.layers.RandomZoom(0.2),
tf.keras.layers.RandomFlip("horizontal"),
tf.keras.layers.RandomContrast(0.2)
])
def preprocess_image(self, image):
image = tf.image.resize(image, [self.image_size, self.image_size])
image = tf.cast(image, tf.float32) / 127.5 - 1
return image
def build_dataset(self, image_paths, labels):
dataset = tf.data.Dataset.from_tensor_slices((image_paths, labels))
return dataset.map(self.load_and_preprocess, num_parallel_calls=tf.data.AUTOTUNE) \
.batch(self.batch_size) \
.prefetch(tf.data.AUTOTUNE)
def load_and_preprocess(self, path, label):
image = tf.io.read_file(path)
image = tf.image.decode_jpeg(image, channels=3)
image = self.preprocess_image(image)
if self.training:
image = self.augmentation(image)
return image, label
Model Architecture
Model Architecture Implementation
class AvianVisionModel(tf.keras.Model):
def __init__(self, num_classes=117):
super(AvianVisionModel, self).__init__()
self.base_model = tf.keras.applications.EfficientNetB3(
include_top=False,
weights='imagenet',
input_shape=(300, 300, 3)
)
# Fine-tuning settings
self.base_model.trainable = True
for layer in self.base_model.layers[:-30]:
layer.trainable = False
# Custom attention mechanism
self.attention = self.build_attention_layer()
# Classification head
self.global_pool = tf.keras.layers.GlobalAveragePooling2D()
self.dropout_1 = tf.keras.layers.Dropout(0.3)
self.dense_1 = tf.keras.layers.Dense(512, activation='relu')
self.batch_norm = tf.keras.layers.BatchNormalization()
self.dropout_2 = tf.keras.layers.Dropout(0.4)
self.output_layer = tf.keras.layers.Dense(num_classes, activation='softmax')
def build_attention_layer(self):
return tf.keras.Sequential([
tf.keras.layers.Conv2D(512, 1, padding='same'),
tf.keras.layers.BatchNormalization(),
tf.keras.layers.Activation('relu'),
tf.keras.layers.Conv2D(1, 1, padding='same'),
tf.keras.layers.Activation('sigmoid')
])
def call(self, inputs, training=False):
x = self.base_model(inputs)
# Apply attention mechanism
attention_weights = self.attention(x)
x = x * attention_weights
# Classification pipeline
x = self.global_pool(x)
x = self.dropout_1(x, training=training)
x = self.dense_1(x)
x = self.batch_norm(x, training=training)
x = self.dropout_2(x, training=training)
return self.output_layer(x)
def get_config(self):
return {"num_classes": self.output_layer.units}
Model Training Process
Training Strategy
- Progressive learning rate reduction
- Mixed precision training
- Gradient accumulation
- Early stopping monitoring
Loss Functions
- Categorical crossentropy
- Label smoothing (0.1)
- Focal loss for class imbalance
- L2 regularization
Training Configuration
class TrainingConfig:
def __init__(self):
self.initial_lr = 1e-4
self.min_lr = 1e-7
self.warmup_epochs = 3
self.weight_decay = 0.01
self.label_smoothing = 0.1
self.mixed_precision = True
def get_callbacks(self):
return [
tf.keras.callbacks.EarlyStopping(
monitor='val_accuracy',
patience=5,
restore_best_weights=True
),
tf.keras.callbacks.ReduceLROnPlateau(
monitor='val_loss',
factor=0.5,
patience=3,
min_lr=self.min_lr
),
tf.keras.callbacks.ModelCheckpoint(
'best_model.h5',
monitor='val_accuracy',
save_best_only=True
)
]
def get_optimizer(self):
return tf.keras.optimizers.Adam(
learning_rate=self.initial_lr,
weight_decay=self.weight_decay
)
Performance Optimization
Model Optimization
- Weight pruning (20% reduction)
- Quantization-aware training
- Layer fusion optimization
- Memory footprint reduction
Inference Optimization
- TFLite conversion
- Int8 quantization
- Operator optimization
- Graph optimization
Model Optimization Implementation
class ModelOptimizer:
def __init__(self, model):
self.model = model
self.input_shape = (300, 300, 3)
def optimize_for_mobile(self):
converter = tf.lite.TFLiteConverter.from_keras_model(self.model)
converter.optimizations = [tf.lite.Optimize.DEFAULT]
converter.target_spec.supported_types = [tf.float16]
converter.target_spec.supported_ops = [
tf.lite.OpsSet.TFLITE_BUILTINS,
tf.lite.OpsSet.SELECT_TF_OPS
]
tflite_model = converter.convert()
return tflite_model
def quantize_model(self):
converter = tf.lite.TFLiteConverter.from_keras_model(self.model)
converter.optimizations = [tf.lite.Optimize.DEFAULT]
converter.target_spec.supported_ops = [tf.lite.OpsSet.TFLITE_BUILTINS_INT8]
converter.inference_input_type = tf.uint8
converter.inference_output_type = tf.uint8
def representative_dataset():
for _ in range(100):
data = np.random.rand(1, *self.input_shape)
yield [data.astype(np.float32)]
converter.representative_dataset = representative_dataset
quantized_model = converter.convert()
return quantized_model
Model Deployment
Mobile Deployment
- TFLite model packaging
- On-device inference optimization
- Memory-efficient loading
- Battery usage optimization
API Integration
- RESTful API endpoints
- Batch prediction support
- Result caching system
- Error handling protocols
Results & Impact
Model Performance Metrics
Classification Performance
- Overall accuracy: 91.23% on test set
- Top-5 accuracy: 98.7%
- F1-score: 0.89 (macro-averaged)
- Inference time: 180ms on mobile devices
Optimization Results
- Model size reduced from 46MB to 12MB
- Inference speed improved by 3.2x
- Memory usage reduced by 72%
- Battery impact reduced by 58%